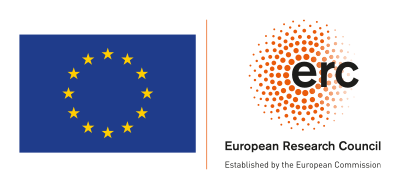
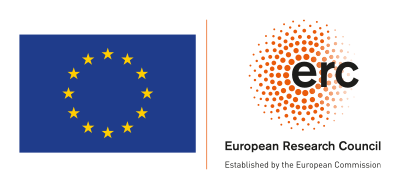
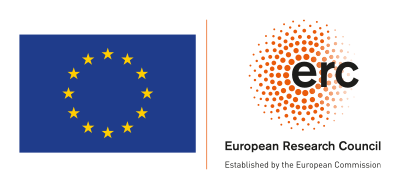
This project has received funding from the European Research Council (ERC) under the European Union’s Horizon 2020 research and innovation programme (grant agreement No 948679).
Project Overview
Summary
The goal of this project is to develop estimation and control strategies for systems where only a (very) limited amount of information (measurements and models) is available. The main motivation to consider these problems are biomedical applications, where such a small amount of available information is often inherent. Examples include hormone concentration measurements when considering thyroidal diseases (which are typically only taken every few days or even weeks) or monitoring the size of a tumor. Estimating the current state of the system and devising appropriate control actions is very challenging in such applications. This is not covered by existing approaches in the literature, necessitating the development of novel methods and tools.
Within this project, we will in particular focus on the following aspects. First, observability of nonlinear systems subject to few (sampled) measurements will be studied and sampling strategies together with suitable nonlinear state estimators will be derived. Second, state estimation and control strategies will be developed for situations with only partial or no model knowledge. Again, this is of intrinsic importance in biomedical applications where often the underlying physical principles are only partially understood or too complex. This necessitates the design of data- and learning-based methods, for which desired guarantees can be given, even in case of few measurements. Third, the developed tools will be extended to large-scale systems, where estimation and control has to be achieved in a distributed fashion.
The successful achievement of the project goals will
- enable estimation and control in systems with very few, sampled measurements,
- constitute a big step towards a holistic data-based systems and control theory,
- result in a new, data-driven, paradigm for the control of large-scale systems, and
- enable the design of systematic, personalized, and optimal control strategies in biomedical applications.
Further Information
Publications
-
(2024): An efficient data-based off-policy Q-learning algorithm for optimal output feedback control of linear systems, Proceedings of the 6th Annual Learning for Dynamics \& Control Conference Weitere Informationen
arXiv: 2312.03451 -
(2024): Sample- and computationally efficient data-driven predictive control, Proceedings of the 22nd IEEE European Control Conference 2024
DOI: 10.23919/ECC64448.2024.10591022
arXiv: 2309.11238 -
(2023): Efficient Off-Policy Q-Learning for Data-Based Discrete-Time LQR Problems, IEEE Transactions on Automatic Control, pp. 1-12
DOI: 10.1109/TAC.2023.3235967 -
(2023): On the design of persistently exciting inputs for data-driven control of linear and nonlinear systems, IEEE Control Systems Letters, vol. 7, pp. 2629 - 2634
DOI: 10.1109/LCSYS.2023.3287133
arXiv: 2303.08707 -
(2023): Data-Based Control of Feedback Linearizable Systems, IEEE Transactions on Automatic Control (Early Access)
DOI: 10.1109/TAC.2023.3249289
arXiv: 2204.01148 -
(2023): An efficient off-policy reinforcement learning algorithm for the continuous-time LQR problem, Accepted for IEEE 62nd Conference on Decision and Control (CDC)
DOI: 10.1109/CDC49753.2023.10384256
arXiv: 2303.17819 -
(2023): Data-driven nonlinear predictive control for feedback linearizable systems, 22nd IFAC World Congress: Yokohama, Japan, July 9-14, 2023. IFAC-PapersOnLine, Vol. 56, Issue 2, pp. 617-624
DOI: 10.1016/j.ifacol.2023.10.1636
arXiv: 2211.06339 -
(2023): Robust Stability of Gaussian Process Based Moving Horizon Estimation, 2023 IEEE 62nd Conference on Decision and Control (CDC), pp. 4087-4093
DOI: 10.1109/CDC49753.2023.10383304
arXiv: 2304.06530 -
(2023): Model predictive control for the prescription of antithyroid agents, Proceedings on Automation in Medical Engineering Weitere Informationen
arXiv: 2307.16690 -
(2023): Notes on data-driven output-feedback control of linear MIMO systems, submitted
arXiv: 2311.17484 -
(2023): Data-based system representations from irregularly measured data, submitted
arXiv: 2307.11589 -
(2023): Identification from data with periodically missing output samples, submitted
DOI: 10.15488/15821 -
(2022): Optimal hormone replacement therapy in hypothyroidism - a model predictive control approach, Frontiers in Endocrinology, 13: 884018
DOI: 10.3389/fendo.2022.884018 -
(2022): Data-Based Moving Horizon Estimation for Linear Discrete-Time Systems, 2022 European Control Conference (ECC), pp. 1778-1783
DOI: 10.23919/ecc55457.2022.9838331
arXiv: 2111.04979 -
(2022): Linear tracking MPC for nonlinear systems part II: the data-driven case, IEEE Trans. Automat. Control, Vol. 67, Issue 9, pp. 4406-4421
DOI: 10.1109/TAC.2022.3166851
arXiv: 2105.08567 -
(2022): Linear tracking MPC for nonlinear systems part I: the model-based case, IEEE Trans. Automat. Control, Vol. 67, Issue 9, pp. 4390-4405
DOI: 10.1109/TAC.2022.3166872
arXiv: 2105.08560 -
(2022): On a continuous-time version of Willems' lemma, IEEE 61st Conference on Decision and Control (CDC), pp. 2759-2764
DOI: 10.1109/CDC51059.2022.9992347 -
(2022): Data-driven distributed MPC of dynamically coupled linear systems, IFAC-PapersOnLine, Vol. 55, Issue 30, pp. 365-370, 25th International Symposium on Mathematical Theory of Networks and Systems (MTNS 2022)
DOI: 10.1016/j.ifacol.2022.11.080 -
(2022): Sample-based observability of linear discrete-time systems, 2022 IEEE 61st Conference on Decision and Control (CDC)
DOI: 10.1109/CDC51059.2022.9993267 -
(2022): Mathematical Modeling and Simulation of Thyroid Homeostasis: Implications for the Allan-Herndon-Dudley Syndrome, Frontiers in Endocrinology, Vol. 13, No. 882788
DOI: 10.3389/fendo.2022.882788 -
(2022): Practical exponential stability of a robust data-driven nonlinear predictive control scheme, Supplementary material - technical report
arXiv: 2204.01150 -
(2022): Robust Data-Driven Moving Horizon Estimation for Linear Discrete-Time Systems, submitted
arXiv: 2210.09017 -
(2021): Data-Based System Analysis and Control of Flat Nonlinear Systems, 60th IEEE Conference on Decision and Control (CDC), pp. 1484-1489
DOI: 10.1109/CDC45484.2021.9683327
arXiv: 2103.02892 -
(2021): Data-driven model predictive control: closed-loop guarantees and experimental results, at–Automatisierungstechnik, vol 69
DOI: 10.1515/auto-2021-0024